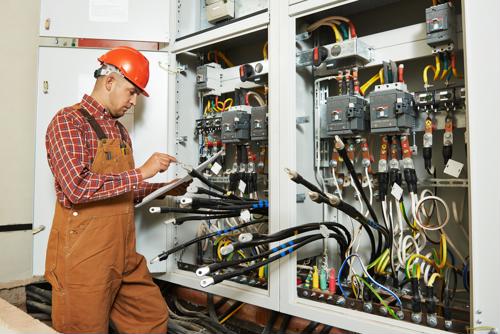
IoT and predictive maintenance: A boon for consumers and enterprises alike
By Max BurkhalterJune 2, 2020
Historically, consumers and enterprises have used preventative maintenance to keep their vehicles and machinery running at peak efficiency. This approach typically relies on predetermined maintenance routines that seek to address common mechanical issues and component failures before they cause lasting damage. However, such a time-based framework has proven to be less cost-effective and more labor-intensive than many realized. In fact, research from The Arc Group found that only 18% of equipment worldwide has failed due to its age - 82% of failures occur randomly and without warning, leading to unplanned downtime, costly repairs and (in some cases) a complete replacement.
Thanks to recent innovations in internet-of-things technologies, companies and end users have been able to take a more proactive stance on equipment maintenance. For example, by outfitting production machinery with internet-connected sensors, manufacturers are able to track performance in real time, forecast potential breakdowns and create more adaptable maintenance schedules. These same benefits are shared by modern vehicles - increased connectivity has provided consumers with more visibility into their cars' performance and relative condition. When mechanical issues are detected, advanced IoT systems can send out automated maintenance alerts to notify vehicle owners that repairs are needed. These capabilities have transformed preventative tasks into a predictive process that leverages historical data and trends to inform better maintenance decisions. But what, exactly, is predictive maintenance and how is it benefiting enterprises and consumers?
Defining predictive maintenance
As noted by the Institute of Industrial and Systems Engineers, predictive maintenance helps eliminate unnecessary upkeep activities through the use of monitoring and diagnostic techniques. In terms of industrial applications, most manufacturers already use IoT sensors to track environmental factors, from ambient temperatures to vibrations and beyond. When all of this data is fed into an intelligent asset management platform - and analyzed using AI and machine learning tools - managers can more accurately predict when specific pieces of equipment will experience a particular maintenance issue. This process benefits enterprises by helping maximize uptime, control repair costs, improve asset longevity and uphold high personal safety standards. More specifically, a 2018 study from PricewaterhouseCoopers found that predictive maintenance can help manufacturing plants:
- Reduce equipment costs by 12%
- Increase uptime by 9%
- Decrease safety, health and quality risks by 14%
- Extend the lifespan of machinery by up to 20%
Of course, taking full advantage of predictive maintenance requires the right data architecture, analytics tools and IT professionals to help make sense of all the raw information being collected. Plant managers must be able to analyze both structured and unstructured data, which may require some upfront investment. This is because most legacy systems and business intelligence platforms weren't designed to handle massive IoT data streams or accommodate the latest advances in data analytics. Like most IT-related initiatives, predictive maintenance requires near-constant optimization and process reform to ensure the best possible outcomes.
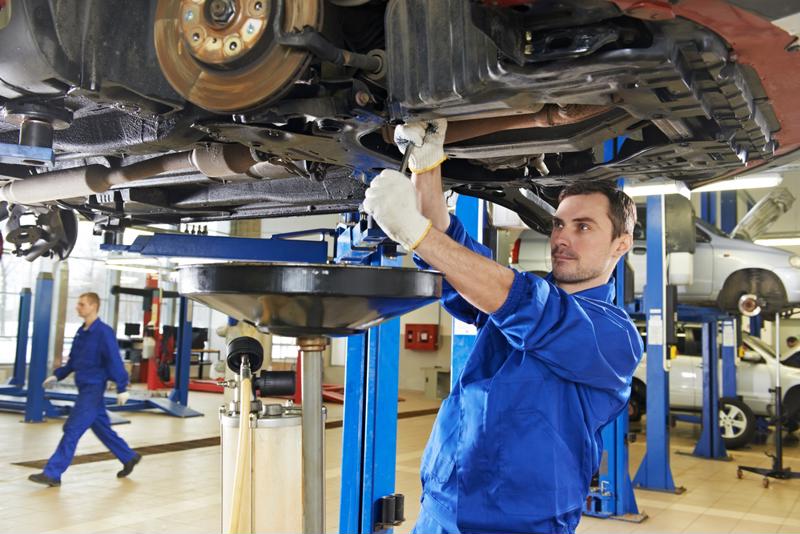
Assessing predictive maintenance use cases
To help business leaders and consumers understand the true potential of predictive maintenance, it's often helpful to highlight specific use cases that are having a meaningful and sustained impact. Since IoT has been adopted by almost every industry, it's easy to locate examples of how this practice is leading to more intelligent and agile IT systems. Here are a few compelling uses cases that every tech decision-maker should consider:
- Preventing power outages: Whether due to intense storms or equipment failures, the utility industry is constantly improving its infrastructure to reduce the frequency and length of power outages. For example, a partnership between IBM and utility companies has led to a variety of innovations that have helped automate asset management and quickly identify the source of power outages. One recent proof of concept involves using predictive analytics to identify trees and other vegetation that may pose a risk to high-power utility lines.
- Reducing motor vehicle accidents: As part of its ongoing efforts to create "zero failure public transport systems," The International Association of Public Transport has been increasingly interested in combining predictive maintenance with smart asset management. One possible application is outfitting all public vehicles (buses, trains, etc.) with IoT sensors to keep transportation officials aware of performance issues and mechanical inefficiencies. Such a move could help reduce breakdowns and create needs-based maintenance routines that will benefit commuters in the long run.
- Protecting sensitive supply chains: As the world grows more interconnected, shipping companies are having to leverage new logistics processes and data analysis tools to safeguard products while in transit, Deloitte noted. This is particularly important for perishable goods, which rely on refrigeration systems when being shipped from the production site to the end consumer. With IoT sensors, supply chain managers can watch over shipping containers and individual orders in real-time, ensuring all HVAC systems are working reliably.
Regardless of the particular application, IoT-powered predictive maintenance requires robust IT infrastructure to ensure critical data is both available and protected from external threats. Perle offers industrial-grade connectivity tools - like our LTE routers and gateways - that allow enterprises to capitalize on digital transformation and aggregate data from hundreds of distinct IoT devices. To learn more, read some of our customers' success stories.